PEP 6305 Measurement in
Health & Physical Education
Topic 8:
Hypothesis Testing
Section 8.1
n
This Topic has 3 Sections.
Reading
n
Vincent & Weir, Statistics in Kinesiology, 4th ed. Chapter
10 “The t Test: Comparing Means from Two Sets of Data”
Objective
n
This topic introduces the basic concepts of hypothesis testing.
n
It is more important to understand these concepts than to memorize
the formulas and application of t tests.
¨
t tests,
the focus of the chapter, are conceptually similar to
Z-tests, except the
statistic (t) is compared to the t distribution instead of
comparing Z to the
normal distribution.
¨
The t distribution is similar the normal
distribution, except for an adjustment for samples with N < 120.
¨
Any hypothesis that can be tested with a t test can also be
tested with analysis of variance (ANOVA), which is our next Topic (ANOVA and t test
conclusions will always be exactly the same). ANOVA is more
general than t tests because ANOVA can test a variety of other
hypotheses.
¨
You can compare two groups (independent t test) or compare
two measures in one group (paired t test) in R Commander, under
Statistics>Means>Independent samples t-test... See the R Help menus for instructions.
Note
n
This topic is fairly long and detailed; please allow yourself
sufficient time to study and complete it.
Overview:
Research Hypothesis vs. Null Hypothesis
n
Recall from
Topic 1 that a research hypothesis is a
potential answer to a
research question about a well-defined problem.
¨
The research hypothesis is developed from a thorough review of the
literature; it is not “a guess.”
·
Ideally, the research hypothesis states the direction (see the
Two-Tailed and One-Tailed Tests
in the following section)
and the size (see the
Effect Size
in the following section) of the hypothetical effect.
¨
The research hypothesis is not tested by statistical
analysis. Statistical analyses test the null hypothesis.
¨
The null hypothesis is written so that if it is true then the research hypothesis
cannot also be true.
·
The null hypothesis directly contradicts the research hypothesis.
Both hypotheses cannot be true.
·
Data that show that the null hypothesis is probably false
(see the
Type I and Type II Errors
in the following section), support (do not "prove") the
research hypothesis.
¨
For example, you compare a treatment group to a non-treatment
(control) group. The research hypothesis is that the treatment will
improve
outcome in the treatment group. The null hypothesis is that the treatment group
will have the same or worse outcome as the control group. 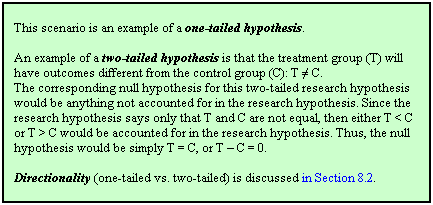
·
In symbolic terms, for this example the research hypothesis is:
Treatment (T) – Control (C) > 0, or T – C > 0. The outcomes in T will be higher
(better) than the outcomes in C. If we rearrange the terms so that T is on the
left of the greater than sign (add C to the right-hand side), then T > C is another way to state the research
hypothesis.
·
The null hypothesis is: T – C ≤ 0. The null hypothesis has to
account for everything not accounted for in the research hypothesis.
Since the research hypothesis is T > C, the null hypothesis has to account for
both T = C and T < C. Thus, T ≤ C.
·
The statements T > C and T ≤ C cannot both be true. T must
either “greater than” or “less than or equal” to C.
·
If a statistical test shows a low
error probability (p ≤ 0.05)
assuming that T ≤ C (the null hypothesis) is true, then T > C (the research hypothesis)
is more likely to be correct and is thus supported.
·
If a statistical test shows a high error probability (p > 0.05)
assuming that T ≤ C (the null hypothesis) is true, then T > C (the research hypothesis)
is less likely to be correct and is thus not supported.
·
Suppose a statistical test of the data show that the error probability of
the null hypothesis is
p = 0.009; this means that the groups are likely to actually have different outcomes.
·
You conclude that the evidence supports the research hypothesis
(treatment improves outcomes).
n
The null hypothesis is tested by comparing an observed value
(computed from sample data) to a statistical distribution.
¨
The investigator designs a study to manipulate the
conditions in order to produce data (evidence) to support the research hypothesis.
¨
The investigator then collects data and computes a statistical
value. This value is the observed value of the statistic.
¨
The investigator identifies the statistical distribution that
provides the sampling distribution of the statistic if the null hypothesis is
true.
¨
The observed statistical value is compared to the statistical
distribution to determine what percent of values are more extreme than the
observed value (see the
Two-Tailed and One-Tailed
Tests in the following section). 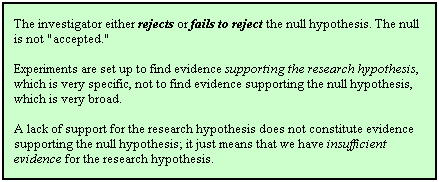
¨
If the error probability of the observed value is
low (<0.05), then the conclusion is that the investigator changed the conditions “significantly”. The
null hypothesis is
rejected, providing support for the research
hypothesis
since it is a more likely explanation for the data than the null.
·
What is the conclusion if the null hypothesis is
not
rejected?
·
The decision to reject or not reject the null hypothesis can never
be made with certainty, although the probability of making an incorrect decision
can be estimated (see the
Type I and Type II
Errors and the
Power and Sample Size
in the following sections).
n
Maybe
another example
would be useful? After reviewing the literature, an investigator
states this research hypothesis: Daily aerobic exercise changes serum cholesterol
level. The
null hypothesis is that daily aerobic exercise has no effect on serum
cholesterol.
¨
The investigator measures serum cholesterol in a sample of
subjects; the mean serum cholesterol is the same in both groups.
¨
The investigator randomly assigns the subjects to two groups.
¨
The first group receives a treatment: aerobic activity for 30
minutes per day for six weeks. The second group receives no treatment.
·
Treatment (exercise or nothing) is the condition manipulated by the investigator
(it is the independent variable). No
other condition is manipulated (all other conditions are the same).
¨
At the end of six weeks, the investigator again measures serum
cholesterol.
¨
The investigator computes a statistical value from the data (such
as to compare the change over time between groups).
¨
The observed value is compared to the distribution of that
statistic which would occur if the serum cholesterol were equal in both groups.
¨
This comparison shows that the observed statistical value is found
to have an error probability of 0.003, which is <0.05.
·
The observed value would only occur 3 times in 1000 such studies
if serum cholesterol were really equal in both groups.
¨
The investigator rejects the null hypothesis.
·
It is more likely that the serum cholesterol levels in the groups
differed.
¨
The analysis thus supports the research hypothesis that daily aerobic
exercise changes serum cholesterol.
·
Aerobic exercise must have affected serum cholesterol because the
subjects were randomly selected and randomly assigned to groups, and all other
conditions during the study were the same for all subjects in both groups.
n
Let's discuss the concepts underlying hypothesis testing in more detail...
Click
to go to the next section (Section 8.2)