PEP 6305 Measurement in
Health & Physical Education
Topic 11:
Reliability
Section 11.2
Click to go to
back to the previous section (Section 11.1)
Intraclass
Correlation
n
The method that we will use to compute a reliability estimate is called
intraclass correlation.
¨
Intraclass correlation uses the MS values that are computed by
analysis of variance (ANOVA).
n
Intraclass correlation can be computed using one-way (simple) ANOVA
or two-way (repeated measures) ANOVA. The
two-way ANOVA will be used in this course.
¨
The one-way ANOVA model is used if differences between the
repeated measures are considered to be error--contrary to intuition,
this is not always the
case.
¨
If the F test of the repeated measures effect is not
significant, the difference in reliability estimates between the one-way and
two-way models is negligible.
¨
You will not be asked to compute reliability using one-way
ANOVA in this course.
n
Calculating reliability is tedious to do manually, but is quite
easy with a program such as LazStats. The approach in this topic will be to use
LazStats to
calculate ANOVA and illustrate the sources of the data.
n
Reliability Example 1 – Random Numbers
¨
This example uses a 4-trial fictional test
administered to 7 fictional individuals. The test scores are random numbers, so
the reliability should be approximately 0.
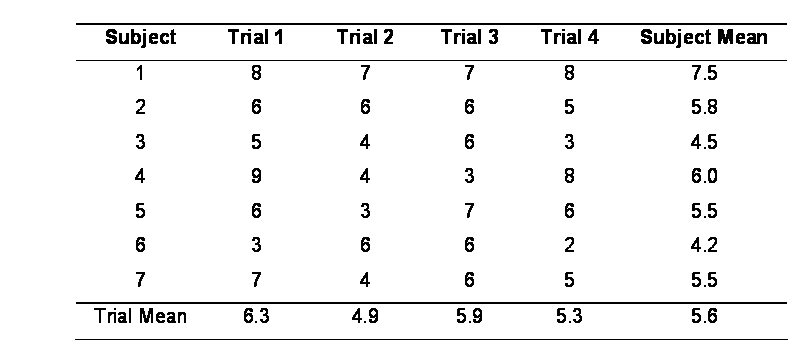
¨
The data set consists of a total of 28 scores, 7 subjects and 4
trials (7 x 4 = 28).
¨
Intraclass reliability will be illustrated using (two-way)
repeated measures
ANOVA of the 28 scores. Here is the resulting ANOVA table:
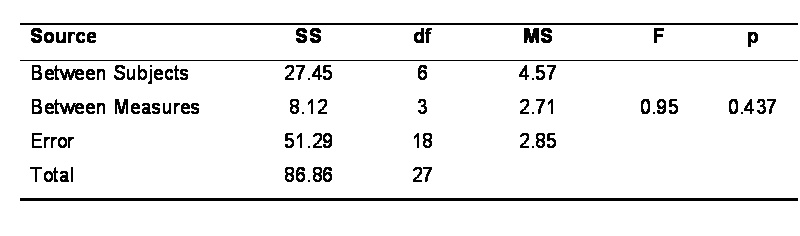
¨
The F value of 0.95 (p = 0.437) is very low, showing that
the variance among the four trials is caused by chance variation: the trials do
not differ.
¨
One equation for calculating intraclass reliability (Rxx)
from a two-way ANOVA is:
Rxx = (MS People - MS Residual) / MS
People = (4.57 - 2.85)/4.56 = 1.72/4.56 = 0.377
(this Rxx is the same as the
intraclass correlation coefficient R2 described by Vincent &
Weir, p. 218, Table 13.4 Model 3,k--it includes only random error, the MS
Residual, and does not include trial-to-trial differences, the MS Between
Measures, as error. Other types of reliability include the trial-to-trial
differences as error, such as when determining how closely raters agree (use
Model 2,k from Table 13.4).
¨
The intraclass reliability of 0.377 is for a test score that is
the mean of all 4 trials. (The effect of number of trials on test
reliability is covered below.)
¨
Note, the 28 scores in this example are random numbers so the
reliability estimate should be low and near 0. The reason that it is not exactly 0 is the
sample size of 7 subjects is very small, so the sampling error is large. If we
had a larger sample (> 50), the sampling error would be smaller and the value
would likely be very close to 0.
¨
Assuming the intraclass reliability was actually 0.377, we can
conclude the following:
·
The proportion of total variance,
measuring true individual differences in whatever was tested, was 0.377 (37.7%).
·
The proportion of total variance
that is measurement error is 0.623 (1.00 – 0.377 = 0.623), or 62.3%.
·
Thus, measurement error is much
larger than true score differences. Reliability is low, and this is a poor test.
n
The purpose of this section is to illustrate the use of R
Commander to
estimate test reliability.
¨
This table provides actual leg strength data for a sample of 6
subjects, each tested 4 times, i.e., there were 4 trials.
Download the ReliabilityEx file from Blackboard or right-click and "Save target as..." to download and save the
ReliabilityEx
file
and import it into
R Commander.
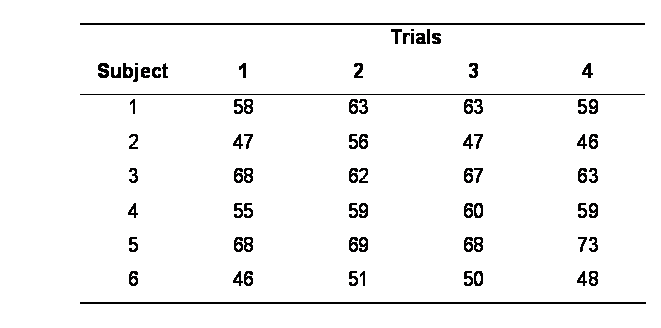
- Enter the following text into the R
Commander Script window:
ezANOVA(data=ReliabilityEx,
dv=.(score), wid=.(subject), within=.(trial), detailed=TRUE)
n
Compare your output to the following:
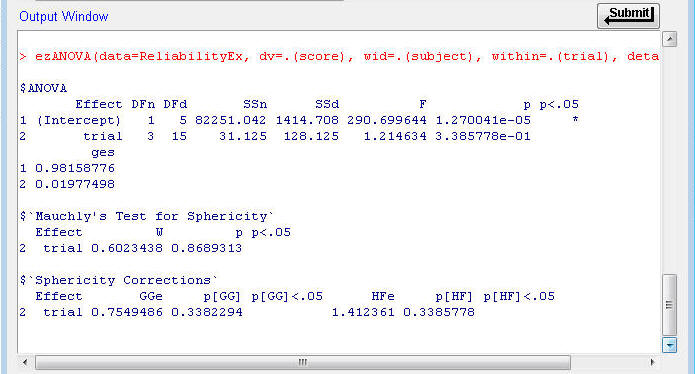
·
Between Persons SS
· The
SS Persons is the df for the denominator of the "Intercept" effect: SS Persons =
1414.708. The Intercept label means the variability of the subjects from the
grand mean across all 4 measures.
· The
MS Subjects = SS Subjects / df = 1414.708 / 5 = 282.88.
·
Between Items F
·
The F value for the between-trials
test is
1.214624 (p = 3.385 x 10-1 = 0.339).
·
Indicates that variation among
the means of the four trials is within chance.
·
Within People Total Variance.
·
The Within Total SS is the sum of the
Between Items SS (31.125) and Residual SS (128.125).
·
The Residual MS = 128.125/15 = 8.542 (This is
close to what you can compute to be the Total Within MS, 8.847, because the trials are not
significantly different; hence, reliability estimates
that do or don't consider trial to trial differences to be error would be essentially the same).
¨
Reliability Estimates
·
To compute this intraclass reliability
coefficient from the ANOVA table values:
Rxx = (MS Subjects - MS Residual)/ MS
Subjects
= 282.88 - 8.542/282.88 = 274.338/282.88 =
0.970
·
This is an intraclass reliability
estimate for a score that is the sum/total of the 4 trials.
¨
Interpretation of the Reliability
coefficient
·
Over 97% of the variance of
strength test is due to true differences in strength among the subjects – some
subjects
are stronger than others and the test measures these differences.
·
Less than 3% of the variance of
the strength test is due to random measurement error.
·
We can conclude that this is a highly reliable test.
Forecasting
Reliability
n
The equation for forecasting test reliability with the ANOVA means
squares.
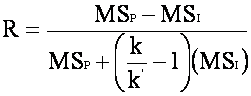
n
The terms are:
¨
MSP = Between People Mean Square.
¨
MSI = Residual Mean Square.
¨
k = number of trials administered.
¨
k' = number of trials for which you want to estimate reliability;
this number will be either greater than or less than k, the number of trials
actually measured.
n
Example: An arm strength test was administered to 207 people and
there were two trials, resulting in the following ANOVA table.
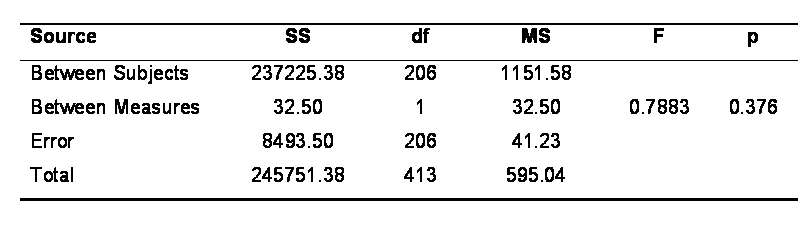
n
Note the following information:
¨
Intraclass Reliability for two trials = 0.964 (How
do we know this?)
¨
Data For Forecast Equation:
·
MSP = Between People
Mean Square = 1151.58.
·
MSI = Residual Mean
Square = 41.23
·
k = number of trials administered
= 2.
¨
Forecasting Reliability: For one trial (k' = 1)
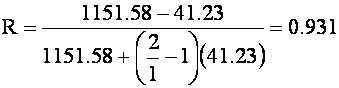
¨
Interpretation - The reliability for the test if only one trial
was administered would be 0.931, slightly lower than for two trials.
¨
Forecasting Reliability - For three trials (k' = 3)
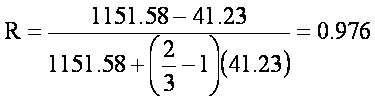
¨
Interpretation - The reliability for the test if three trials were
administered, the reliability would be 0.976, slightly higher than for two
trials (0.964).
n
Application of Forecasting
¨
The forecasting method can be used to develop a testing protocol.
¨
In the example just illustrated, the reliability of a 2-trial test
was very high. This analysis showed that just using a single trial would result
in only a small loss in accuracy.
¨
The method can also be used to determine how many additional
trials would be required to improve reliability.
n
The Spearman-Brown prophecy formula is a second method that can be
used to forecast test reliability.
¨
This method estimates the increase in test reliability for a test
that has been lengthened by k times (k = 2, the test is twice as long, k = 0.5,
the test is half as long, etc.).
¨
The reliability of test can be estimated for a longer test. The
Spearman-Brown equation is:
where
r1,1 is the computed reliability of the test, k is times the test is
lengthened, and rkk is the estimated reliability for the test
lengthened k times.